Discover the path to becoming a data scientist: Key steps and insights on how do I become a data scientist. Your journey to a rewarding career in data science starts here. Data science is a dynamic and multidisciplinary field that has gained tremendous prominence in recent years.
It revolves around the extraction of valuable insights and knowledge from vast and complex datasets using a combination of statistical, computational, and domain-specific expertise. Data scientists play a crucial role in transforming raw data into actionable information, making data-driven decisions, and solving real-world problems across various industries.
At the heart of data science is data exploration and analysis. Data scientists employ a range of tools and techniques to clean, preprocess, and visualize data, uncovering patterns, trends, and correlations that can inform business strategies or scientific discoveries.
They often work with programming languages like Python and R, utilizing libraries and frameworks like pandas, NumPy, and scikit-learn to manipulate and model data effectively.
Machine learning is a central component of data science, where algorithms and models are trained to make predictions, classify data, or automate tasks.
Supervised learning, unsupervised learning, and reinforcement learning are among the key paradigms within machine learning that data scientists utilize to build predictive models. Deep learning, a subset of machine learning, has gained prominence in tasks such as image and speech recognition.
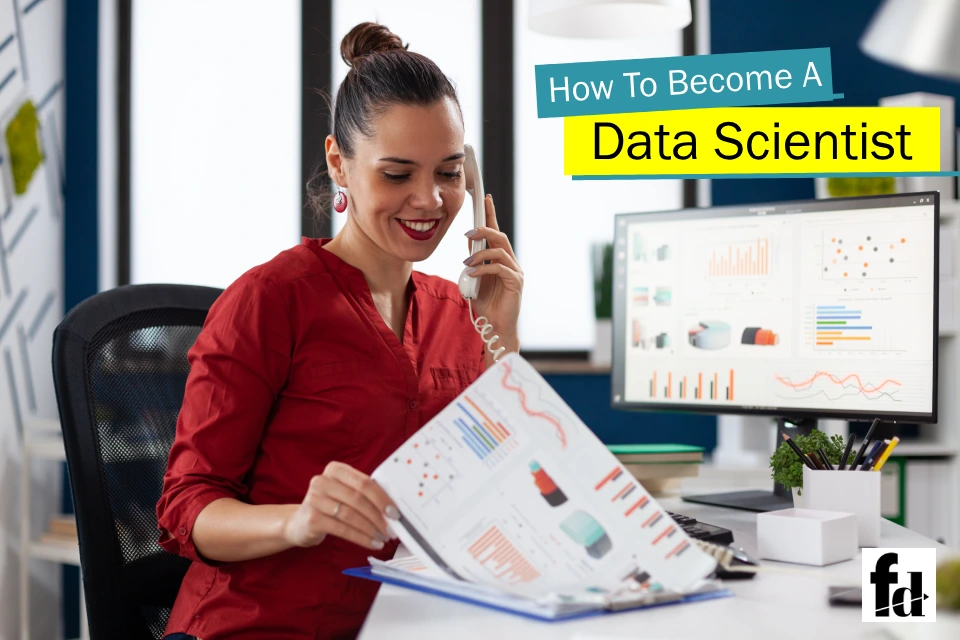
Why Does Data Science Matters?
Data science has become ubiquitous in today’s world. Companies worldwide are increasingly turning to data science to tackle a wide range of challenges. This trend has created a highly favorable job market for data scientists, with competitive salaries and opportunities aplenty.
Chances are, if you’re reading this article, you’re intrigued by the idea of becoming a data scientist. In the following paragraphs, we’ll guide you through the essential steps to embark on your journey in the data science field.
We’ll also introduce you to an effective and appealing approach to gaining the necessary skills to kickstart your data science career. We’ve even included data scientists in our list of top analytics careers, and you can explore other exciting roles in our comprehensive article. So, let’s dive into the world of data science and discover how you can be a part of this thriving field.
Data science is applied in a multitude of domains, from finance and healthcare to marketing and transportation. For example, in healthcare, data scientists analyze patient records and medical images to improve diagnosis and treatment plans, while in finance, they develop algorithms for fraud detection and risk assessment. In marketing, data science helps optimize advertising campaigns and customer segmentation, leading to more effective marketing strategies.
Ethical considerations are paramount in data science, given the potential for bias, privacy breaches, and unintended consequences when working with sensitive data. Responsible data handling and ethical decision-making are crucial aspects of the profession, and data scientists often collaborate with ethicists and legal experts to ensure that their work respects societal norms and regulations.
Data science is a rapidly evolving field with far-reaching implications for how businesses and organizations operate in the data-driven age. It combines data analysis, machine learning, and domain knowledge to extract valuable insights from data and make informed decisions that can drive innovation and improve processes across diverse sectors of the economy.
As the volume and complexity of data continue to grow, the role of data scientists in harnessing its potential will only become more critical.
What Does a Data Scientist Do?
A data scientist is a skilled professional who specializes in extracting valuable insights and knowledge from data, typically large and complex datasets. Their role is crucial in today’s data-driven world, where businesses and organizations rely on data-driven decision-making to gain a competitive edge and solve complex problems.
Data scientists play a critical role in shaping their team’s inquiries and devising data-driven answers. They’re responsible for crafting predictive models to support theories and forecasts. In essence, they guide the process of asking the right questions and finding data-backed solutions.
A data scientist might do the following tasks on a day-to-day basis:
- Find patterns and trends in datasets to uncover insights
- Create algorithms and data models to forecast outcomes
- Use machine learning techniques to improve the quality of data or product offerings
- Communicate recommendations to other teams and senior staff
- Deploy data tools such as Python, R, SAS, or SQL in data analysis
- Stay on top of innovations in the data science field
One of the primary responsibilities of a data scientist is data collection and preprocessing. They gather data from various sources, which can include databases, sensors, web scraping, or even social media.
This data can be structured or unstructured, and data scientists must clean, format, and organize it to make it suitable for analysis. Data preprocessing ensures that the data is accurate and consistent, reducing the risk of erroneous conclusions.
Data exploration and analysis are core tasks for data scientists. They use statistical techniques and data visualization tools to uncover patterns, trends, and relationships within the data.
This exploration phase helps organizations better understand their data and make informed decisions. Data scientists often work closely with domain experts to ensure that their analysis is relevant and aligns with the goals of the business or project.
Machine learning plays a significant role in the work of data scientists. They build and train machine learning models to predict future outcomes, classify data, or automate tasks.
Supervised learning, where models are trained on labeled data, and unsupervised learning, which discovers patterns in unlabeled data, are common techniques. Deep learning, a subset of machine learning, is used for complex tasks like image recognition and natural language processing.
Communication is a vital skill for data scientists. They must effectively convey their findings and insights to non-technical stakeholders, such as executives or decision-makers. Visualization techniques, reports, and presentations are essential tools for presenting complex technical information in a clear and understandable manner.
Data scientists are responsible for maintaining the integrity and security of the data they work with. They must adhere to ethical guidelines and data privacy regulations to ensure that sensitive information is handled appropriately and that the results of their analysis are trustworthy.
Data scientists are professionals who excel at collecting, cleaning, analyzing, and interpreting data to solve real-world problems and inform strategic decisions. Their multifaceted role combines expertise in data manipulation, statistical analysis, machine learning, and effective communication to unlock the potential of data for organizations across various industries.
Fundamentally, data scientists dive into the world of data to uncover valuable insights that can drive action. In the corporate landscape, their role spans various teams, where they play a pivotal role in establishing a strong analytical foundation.
This involves crafting plans to collect, acquire, and scrub data from diverse origins. Once the data is well-organized and explored, they can construct innovative solutions and convey their discoveries to the broader organization.
Collaboration is a cornerstone of a data scientist’s work. They frequently team up with a range of experts, such as data engineers, analysts, IT architects, app developers, and business leaders, to ensure that data-driven insights align with the company’s goals and strategies.
In short, data scientists bridge the gap between raw data and actionable intelligence, fostering informed decision-making across the business landscape.
Data Analyst vs Data Scientist: What’s the Difference?
To put it simply, these roles deal with data but have distinct focuses and responsibilities:
Data analysts:
Their primary task involves deciphering existing data to offer practical insights. They usually employ more straightforward models and tools in their work.
Think of data analysts as the detectives of the data world. Their main job is to examine existing data to find useful insights and trends. It’s a bit like sifting through a pile of clues to solve a mystery. They often use simpler tools and models to do this. Imagine them as the ones who tell you what happened based on the available evidence.
Data scientists:
Their role extends further, often defining the questions that need addressing. They leverage advanced tools and models, like machine learning algorithms, to extract insights from the data. In essence, data scientists dig deeper and cast a wider net to uncover valuable information.
Data scientists take things a step further. They not only look at existing data but also figure out what questions need to be asked in the first place. They’re like the architects of data analysis.
Data scientists use more advanced tools and techniques, including fancy stuff like machine learning algorithms. They dive deep into data and can predict future trends, making them a bit like fortune tellers for businesses.
In a nutshell, while both deal with data, data analysts focus on what’s already there and provide insights, whereas data scientists dig deeper, ask new questions, and use more complex methods to provide a broader range of insights, often predicting what might happen in the future.
Why Become a Data Scientist?
There are plenty of compelling reasons to consider a career in data science. It’s a field that offers both intellectual stimulation and substantial rewards. Data scientists thrive in an ever-evolving landscape, where staying at the forefront of technology and trends is part of the job.
So, if you’re naturally curious, have a knack for analyzing information, and relish the thought of working with data and cutting-edge technology, a path in data science might be your calling.
The job market for talented data scientists is thriving. According to statistics from Statista, the big data market is set to grow significantly, projected to reach $103 billion by 2027, up from $70 billion in 2022. The U.S. Bureau of Labor Statistics predicts an impressive 36% surge in data science job opportunities between 2021 and 2031, far surpassing the national average of 4%.
This booming demand is reflected in the rankings. For instance, U.S. News & World Report lists data scientist as the 3rd best job in technology, 6th in STEM jobs, and 6th overall. Glassdoor also rates it as the 3rd best job in America for 2022.
Still not convinced? Consider the average data scientist salary, with a median of $102,000 per year. It’s evident that the demand for data scientists comes with an enticing paycheck, making it a compelling choice for those seeking a fulfilling and financially rewarding career.
Here are common reasons why you should become a data scientist:
● Data science is all about solving puzzles and uncovering hidden insights within data. If you enjoy solving complex problems, playing with data, and finding patterns that others might miss, this field can be incredibly fulfilling. It’s like being a detective in the world of numbers and information.
● Data science is in high demand across various industries. Companies of all sizes, from tech giants to healthcare providers and retailers, are hungry for data-driven insights to make informed decisions. This demand means there are plenty of job opportunities and job security in the field.
● The data science field is dynamic and ever-evolving. It’s not stagnant; it constantly adapts to new technologies and methodologies. If you love learning and staying on the cutting edge of technology, data science keeps you intellectually engaged.
● Financially, data science can be quite rewarding. The salaries in this field are competitive, with the potential for substantial earnings as you gain experience and expertise. So, it’s a career path that not only satisfies your intellectual curiosity but also your wallet.
● Data science has a positive impact on the world. You can work on projects that contribute to scientific advancements, healthcare improvements, environmental conservation, and much more. It’s a chance to use your skills to make a difference.
● This means, becoming a data scientist offers a blend of intellectual challenge, job security, continuous learning, financial rewards, and the opportunity to make a meaningful impact. It’s a profession that appeals to both the analytical minds and those who want to create a better future through data-driven insights.
What Qualifications do Data Scientists Need?
The question of whether you need a degree to become a data scientist sparks some debate. While many professionals have found their way into the field through alternative paths, a university degree can certainly be advantageous.
When you look at graduate programs and job listings, you’ll often find that they prefer candidates with qualifications in fields like computer science, data science, mathematics, statistics, engineering, and even physics. However, there are some programs that are willing to train individuals with any degree of background to become proficient data scientists.
Speaking of skills, we’ll delve into those in more detail shortly, but having a working knowledge of programming languages like Python, R, SQL, and/or Julia can be quite beneficial.
On the flip side, some folks have charted their own course by engaging in self-directed learning. They pick up the necessary skills and experience at their own pace and shine during interviews. For example, you can explore our Data Scientist with Python track to acquire all the skills needed to kickstart a data science career.
If you want to demonstrate your data science expertise to potential employers, consider getting certified as a data scientist through platforms like DataCamp. It’s a way to show that you’re well-equipped for the job, regardless of your academic background.
Qualifications and Skills of Data Scientists:
While there are various paths to becoming a data scientist, a combination of educational qualifications, technical skills, and domain expertise can significantly enhance your prospects in this exciting and dynamic field. Continuous learning and adaptability are key to a successful career in data science.
Data science is a field where qualifications can vary, but there are some key qualifications and skills that can help you excel in this role.
- Educational Background: Many data scientists have at least a bachelor’s degree, and a substantial number have advanced degrees like a master’s or Ph.D. While degrees in fields like computer science, data science, mathematics, statistics, engineering, or physics are often preferred, data science is interdisciplinary, and professionals from various academic backgrounds can thrive in this field.
- Programming Skills: Proficiency in programming languages like Python and R is almost a must for data scientists. These languages are widely used for data analysis, machine learning, and data visualization. Having a working knowledge of SQL for database management can also be very beneficial.
- Statistics and Mathematics: A strong foundation in statistics and mathematics is vital for data scientists. You’ll need these skills to understand data distributions, make inferences, and build statistical models.
- Machine Learning: Familiarity with machine learning algorithms and techniques is increasingly important in data science. This includes supervised and unsupervised learning, deep learning, and reinforcement learning.
- Data Manipulation: You should be skilled in data manipulation and cleaning. This involves using tools like pandas and NumPy in Python to preprocess and prepare data for analysis.
- Data Visualization: The ability to create clear and compelling data visualizations is crucial for communicating your findings effectively. Tools like Matplotlib and Seaborn in Python or ggplot2 in R can help with this.
- Big Data Tools: Depending on the scale of data you work with, knowledge of big data tools and technologies like Hadoop, Spark, or distributed computing can be advantageous.
- Domain Knowledge: Having expertise in the specific industry you work in can be a significant asset. Understanding the nuances and challenges of a particular domain can help you derive more meaningful insights from data.
- Communication Skills: Data scientists must be able to explain their findings to non-technical stakeholders. Good communication skills, both written and verbal, are essential to convey complex insights in a clear and understandable manner.
- Continuous Learning: The data science field evolves rapidly. Staying updated with the latest tools, techniques, and trends is essential to remain competitive and effective in your role.
What Skills Do Data Scientists Need?
Here are the most common data scientist skills you must know if you are planning to become a data scientist:
Hard Skills:
These hard skills provide the technical expertise needed to excel as a data scientist. Mastery of these skills empowers professionals to extract insights, build models, and solve complex problems in the data-driven world.
These are the technical skills you’ll need to develop to become a fully-fledged data scientist:
- Python
- R
- Statistics and math
- SQL and NoSQL
- Data visualization
- Machine learning
- Deep learning
- Natural language processing
- Big data
- Cloud computing
Soft Skills:
These are now usually referred to as ‘power skills’ or ‘human skills,’ and they are the cross-functional skills that aren’t job specific but are still necessary. For a data scientist, these include:
- Communication
- Storytelling
- Critical thinking
- Business acumen
- Problem-solving
- Teamwork
Hard skills of data scientists explained:
● Python: Python is a versatile and widely-used programming language in data science. It’s essential for data manipulation, analysis, and machine learning.
● R: R is another programming language popular among data scientists, especially for statistical analysis and data visualization.
● Statistics and Math: A solid understanding of statistics and mathematics is fundamental for interpreting data, making informed decisions, and building accurate models.
● SQL and NoSQL: Knowledge of structured query language (SQL) for managing relational databases and NoSQL databases is crucial for data retrieval and storage.
● Data Visualization: Data visualization skills help in effectively communicating insights through charts, graphs, and dashboards, making complex data more accessible.
● Machine Learning: Proficiency in machine learning techniques is essential for predictive modeling, classification, and regression tasks.
● Deep Learning: Deep learning, a subset of machine learning, involves neural networks and is particularly useful for complex tasks like image recognition and natural language processing.
● Natural Language Processing (NLP): NLP focuses on enabling machines to understand, interpret, and generate human language, making it vital for text analysis and chatbots.
● Big Data: Understanding how to handle and process large volumes of data efficiently is critical in a data scientist’s role.
● Cloud Computing: Knowledge of cloud platforms like AWS, Azure, or Google Cloud is valuable for scalable data storage, processing, and deployment of data science solutions.
Soft skills of data scientists explained:
● Communication: Effective communication is vital for data scientists to convey complex findings and insights to both technical and non-technical stakeholders. It ensures that data-driven recommendations are understood and acted upon.
● Storytelling: Storytelling skills enable data scientists to craft compelling narratives around their data-driven insights. A well-told data story can make complex information more relatable and actionable.
● Critical Thinking: Critical thinking involves the ability to analyze situations, question assumptions, and make informed decisions. In data science, it’s crucial for evaluating data quality, model performance, and problem-solving.
● Business Acumen: Understanding the business context in which data science operates is essential. Data scientists should align their work with the organization’s goals and objectives to provide value effectively.
● Problem-Solving: Problem-solving skills are at the heart of data science. Data scientists use their analytical abilities to tackle real-world challenges and find innovative solutions using data.
● Teamwork: Data science projects often involve collaboration with cross-functional teams, including data engineers, analysts, and business stakeholders. Strong teamwork and interpersonal skills facilitate effective cooperation and knowledge sharing.
What is an Average Data Scientist Salary?
The average data scientist salary in the United States typically ranged from $80,000 to $180,000 per year. However, salaries can vary significantly depending on factors such as location, experience, education, and the specific industry or company.
When it comes to data scientist salaries, the numbers can vary depending on the sources you look at. For instance, in the United States, Glassdoor reports an average annual salary of around $117,212, based on a dataset of 18,000 salaries. On the other hand, PayScale estimates it at approximately $97,318 per year, drawing from a pool of 7,280 salary profiles.
Now, if we talk about data science managers, they tend to be the top earners in this field, with annual salaries ranging from $113,000 to $230,000.
For data scientists and data engineers, their earning potential is usually quite similar. Data scientists can expect to earn between $82,000 and $167,000 per year, while data engineers typically fall in the range of $76,000 to $166,000 annually.
Keep in mind that the size of the company you work for also plays a role in determining your salary. Larger companies with over 1,000 employees often offer median salaries ranging from $90,000 to $110,000 per year.
Medium-sized companies, with 26 to 1,000 employees, usually offer around $80,000. Meanwhile, smaller companies and startups with 2 to 25 team members may provide salaries in the ballpark of $60,000 per year.
How Do I Become A Data Scientist
We understand that you’re probably excited to kickstart your journey towards becoming a data scientist. But you might be wondering, “Where do I even begin?” Well, don’t worry. We’ve got you covered. Below, we’ve outlined 8 essential steps that can help you start your journey to becoming a data scientist, even if you’re starting from scratch.
Now, it’s important to note that the specific requirements for becoming a data scientist can vary based on various factors. However, these steps we’re about to share are some of the most commonly recommended ones to get you started on the right track.
8 steps to become a data scientists:
- Learn data wrangling, data visualization, and reporting
- Work on your statistics, math, and machine learning skills
- Learn to code
- Understand databases
- Learn to work with big data
- Get experience, practice, and meet fellow data scientists
- Take an internship or apply for a job
- Follow and engage with the community
How do I become a data scientist:
1. Learn data wrangling, data visualization, and reporting
As you progress on your journey to becoming a data scientist, you’ll frequently come across vast and intricate datasets. Making sense of this wealth of information, both for your own understanding and for sharing insights with others, is a vital skill.
This is where data wrangling skills become invaluable. Data wrangling is the art of cleaning, organizing, and transforming raw data into a format that’s ready for analysis and can help you draw meaningful conclusions.
Now, there are various tools available for data wrangling, but one of the most popular choices is the pandas library in Python. If you’re interested, you can explore our handy cheat sheet on data wrangling in Python using pandas for more insights and tips.
When it comes to effectively presenting your data and making a real impact, you’ll need to master the art of reporting and data visualization. There’s a plethora of data visualization tools out there, each offering its unique strengths and capabilities. These tools provide you with a range of options to choose from, allowing you to create visualizations that best suit your specific goals and objectives.
● Data Wrangling: This is like preparing ingredients for a recipe. Data wrangling involves cleaning, organizing, and structuring raw data so that it’s ready for analysis. You’ll remove inconsistencies, deal with missing values, and transform data into a format that’s easy to work with. Think of it as the process of getting your kitchen ready before you start cooking – you want everything to be in order.
● Data Visualization: Once your data is prepared, it’s time to visualize it. Think of data visualization as the art of presenting your dish in an appealing way. You’ll use charts, graphs, and other visual tools to convey complex information in a clear and understandable manner. Just as a chef presents a beautifully plated dish, data scientists create visualizations that help others grasp insights at a glance.
● Reporting: After analyzing the data and drawing meaningful conclusions, you need to communicate your findings effectively. This is similar to sharing your recipe with others. Reporting involves creating reports, presentations, or narratives that convey your insights to stakeholders, whether they are colleagues, clients, or decision-makers. It’s about telling the story behind the data and guiding others through your analysis process.
2. Work on your statistics, math, and machine learning skills
While having a formal degree in fields like calculus, linear algebra, and statistics isn’t an absolute requirement, having a functional understanding of these subjects is crucial. It’s worth considering your specific goals when diving into these areas.
For instance, gaining proficiency in calculus can empower you to create optimization algorithms for machine learning, although it’s worth noting that both Python and R offer functions that handle the calculus side for you.
To illustrate, understanding concepts like gradient descent allows you to measure how a function’s output changes when you tweak its inputs, a skill that can be invaluable for refining machine learning models.
If you’re looking to sharpen your linear algebra skills, you might find our Linear Algebra for Data Science in R course helpful. It covers working with vectors and matrices, solving matrix-vector equations, conducting eigenvalue/eigenvector analysis, and employing principal component analysis for dimension reduction, all of which are highly relevant to applied mathematics and data science.
Similarly, our Intermediate Statistical Modeling in R course delves into essential machine learning statistics topics, providing valuable insights and skills to help you make strides in critical areas.
● Statistics: Think of statistics as the toolkit that helps you understand and interpret data. You’ll learn about concepts like probability, distributions, hypothesis testing, and regression analysis. This knowledge allows you to uncover patterns, relationships, and trends within datasets. It’s like having the ability to read the “language” of data, helping you make informed decisions based on evidence.
● Mathematics: Mathematics serves as the theoretical framework for data science. You’ll delve into concepts like linear algebra, calculus, and optimization. These mathematical foundations are essential for understanding machine learning algorithms and building predictive models. Just as a chef needs to understand the science of cooking, a data scientist must grasp the mathematical principles behind the tools they use.
● Machine Learning: Machine learning is like having a set of specialized kitchen appliances for data analysis. It involves the study of algorithms and models that enable computers to learn and make predictions from data. You’ll explore techniques like decision trees, neural networks, and clustering. This skill empowers you to develop predictive models and uncover valuable insights from data automatically.
3. Learn to code
In our article covering the top programming languages for data scientists, we take a closer look at some of the most valuable languages to consider mastering right now. When you step into the world of data science, two standout choices are Python and R. They’re incredibly versatile and widely used, making them must-learn languages for data enthusiasts.
But data work also involves databases, so SQL becomes another essential programming language. The good news is that it’s relatively straightforward, especially once you’ve gained proficiency in Python and R.
Now, when you’re ready to expand your coding repertoire, Julia is an excellent option. After becoming familiar with Python, R, and SQL, Julia offers a language designed from the ground up for data science, boasting speed and user-friendliness. It’s especially handy when dealing with substantial datasets, although for very large data sets, you might eventually explore other languages.
Java is another contender in the data science arena. This open-source language is known for its efficiency and performance. Java Virtual Machines provide a robust framework for popular big data tools like Hadoop, Spark, and Scala, making it a valuable addition to your skillset.
For those dealing with exceptionally large datasets, languages like Scala, C/C++, JavaScript, Swift, Go, MATLAB, and SAS are worth considering. They offer specialized capabilities that can be particularly advantageous in managing and analyzing extensive data collections.
● Coding Proficiency: Learning to code is like acquiring the language of data science. You’ll need to become proficient in programming languages such as Python, R, or even Julia. These languages are the tools you’ll use to manipulate, analyze, and visualize data. Just as a chef uses knives and utensils in the kitchen, a data scientist uses coding to work with data.
● Data Manipulation: Coding allows you to manipulate and transform data to suit your analysis needs. You’ll write scripts and programs to clean messy data, perform calculations, and create data structures. It’s like having the ability to customize your ingredients and recipes for different dishes.
● Analysis and Modeling: With coding skills, you can implement complex statistical analyses and machine learning algorithms. You’ll write code to build predictive models, explore patterns in data, and make data-driven decisions. This is akin to creating unique culinary creations by combining various ingredients and cooking techniques.
● Automation: Coding also enables automation in data science tasks. You can write scripts to automate repetitive processes, saving time and ensuring consistency in your analyses. It’s like having a kitchen assistant to help with prep work.
4. Understand databases
Let’s revisit the importance of SQL, as it’s a crucial tool for data scientists. When it comes to storing structured data in a speedy and efficient manner, relational databases are the go-to choice. SQL plays a pivotal role in collecting, organizing, and managing data in this context.
SQL empowers you to work with structured data effectively. It allows you to perform tasks like querying databases, handling data wrangling, preparation, and experimentation, and much more.
What’s particularly handy is that SQL often goes hand-in-hand with Python, thanks to libraries like SQLite, PostgreSQL, and MySQL. These libraries facilitate seamless connections between various data sources, giving you the flexibility to work with data more efficiently.
● Data Storage: Databases serve as the digital storage units for your data, similar to how a pantry stores ingredients in a kitchen. You’ll need to understand the different types of databases, with a particular focus on relational databases. These databases use tables to store structured data in a way that can be easily queried and retrieved.
● SQL Proficiency: SQL (Structured Query Language) is the language used to interact with databases. It’s like the recipe book that guides you in preparing dishes. You’ll need to become proficient in SQL to write queries that retrieve, update, and manipulate data within databases. SQL is the bridge that connects you to your data, allowing you to extract the information you need.
● Data Retrieval: Just as a chef knows how to access ingredients in the pantry, a data scientist must know how to retrieve data from databases. You’ll use SQL queries to pull specific datasets that are relevant to your analysis or modeling tasks.
● Data Integrity: Ensuring data integrity is critical in databases. Similar to maintaining the freshness and quality of ingredients, you’ll learn how to maintain the accuracy and consistency of data within databases. This involves techniques like data validation and constraints.
● Database Management Systems: You’ll encounter various database management systems (DBMS), such as MySQL, PostgreSQL, Oracle, and more. Understanding the features and capabilities of these systems is crucial, as they provide the tools for efficient data storage and retrieval.
5. Learn to work with big data
As a data scientist, you’ll frequently find yourself working with vast datasets. In today’s data-driven age, where information is generated in abundance, these datasets keep getting larger and more challenging to handle, from collection to maintenance and processing.
However, it’s from these colossal datasets that skilled data scientists can unearth valuable, detailed insights. This is where proficiency in using cloud platforms like AWS, Microsoft Azure, and Google Cloud comes into play.
These platforms offer a treasure trove of tools and resources to support your data science career. Tools like Apache Spark are indispensable for managing, analyzing, and even running machine learning on big data.
To dive into the fundamentals of big data and get a grip on tools like PySpark, you can explore our course. It covers the essentials you need to navigate and analyze large datasets effectively, a skill that can elevate your data science career.
● Handling Large Volumes: Big data refers to datasets that are so massive and complex that they can’t be easily processed using traditional methods. Learning to work with big data is like upgrading your kitchen to handle large banquets. You’ll need tools and techniques to manage and analyze vast amounts of data.
● Scalable Solutions: Traditional data analysis tools and methods may not suffice when dealing with big data. You’ll need to explore scalable solutions, like cloud platforms (e.g., AWS, Azure, Google Cloud), and distributed computing frameworks (e.g., Hadoop, Spark). These tools are like industrial-sized kitchen equipment that can process data on a grand scale.
● Data Processing: Big data often requires parallel processing to crunch through enormous datasets efficiently. You’ll learn how to break down tasks into smaller chunks, distribute them across multiple machines, and then combine the results. It’s like having multiple chefs working together to prepare a large feast.
● Real-time Analysis: Big data may include real-time or streaming data. You’ll acquire skills to analyze data as it’s generated, making immediate decisions and predictions. This is similar to cooking dishes in real-time as orders come into a restaurant kitchen.
● Data Storage: With big data, you’ll explore various storage solutions, including distributed file systems (e.g., Hadoop HDFS) and NoSQL databases (e.g., MongoDB, Cassandra). These are like specialized storage areas in your kitchen designed to accommodate a large volume of ingredients.
6. Get experience, practice, and meet fellow data scientists
Like any career, becoming a proficient data scientist requires ample experience and practice. The good news is, there are numerous ways to immerse yourself in the data science community, work on projects, and hone your skills.
One valuable resource is DataCamp Workspace, which offers a collaborative, cloud-based notebook. It’s your ticket to not just learning about data science but actually diving into it headfirst.
Workspace facilitates data analysis, teamwork, and sharing insights. Plus, it comes equipped with built-in datasets, meaning you can start exploring and analyzing data in a matter of minutes.
You can apply your newfound knowledge to a variety of data science projects. These projects provide you with the opportunity to tackle real-world challenges right from your web browser. It’s a practical way to put your skills to the test and gain hands-on experience.
● Hands-On Experience: Just as a chef becomes better by cooking a variety of dishes, a data scientist becomes proficient by working on real-world data projects. Seek opportunities to apply your skills to actual data analysis tasks. This practical experience helps you understand the nuances of working with different datasets and solving specific problems.
● Project Portfolio: Building a portfolio of data science projects is akin to creating a chef’s portfolio of signature dishes. It showcases your capabilities and demonstrates your ability to solve practical challenges using data. Prospective employers often look for a strong portfolio when considering candidates for data science roles.
● Collaboration: Collaborating with fellow data scientists is like joining a culinary team in a busy kitchen. You’ll learn from others, share insights, and gain exposure to diverse perspectives and approaches. Collaborative projects also allow you to tackle more complex challenges and expand your skill set.
● Networking: Just as chefs attend cooking events and culinary workshops to network with peers, data scientists benefit from connecting with others in the field. Attend data science meetups, conferences, webinars, and online communities. These interactions can lead to valuable insights, job opportunities, and professional growth.
● Continuous Learning: Data science is a rapidly evolving field, much like the culinary world constantly innovates. Engaging with fellow data scientists and staying active in the community helps you keep up with the latest trends, tools, and techniques. It’s a way to ensure that your skills remain current and competitive.
7. Take an internship or apply for a job
After you’ve honed the skills we’ve discussed earlier, it’s time to put them to work in a professional setting. When you’re confident that you possess the necessary data scientist skills to meet the demands of the role, you can start exploring opportunities like internships or jobs.
For an in-depth guide on how to navigate the process of applying for data science positions and stand out from the competition, check out our comprehensive resource. Building a robust portfolio showcasing your diverse skill set is often a requirement. It’s crucial to prepare thoroughly for data scientist interviews to maximize your chances of success.
● Hands-On Experience: Landing an internship or job in data science is like getting a seat in a restaurant’s kitchen to learn from experienced chefs. It provides you with valuable hands-on experience in applying your data science skills to solve actual problems for a company or organization.
● Skill Validation: Working in a professional role allows you to validate your skills and knowledge. You’ll apply what you’ve learned in a practical context, demonstrating your ability to contribute to real projects. It’s like a chef showcasing their culinary expertise by preparing dishes for paying customers.
● Networking: An internship or job in data science exposes you to a network of professionals in the field. You’ll collaborate with colleagues, mentors, and supervisors who can offer guidance and mentorship. Building these relationships can open doors to further opportunities in the data science community.
● Portfolio Enhancement: The projects you work on during an internship or job become valuable additions to your data science portfolio. These real-world projects demonstrate your ability to apply data science techniques to address business challenges, making you more attractive to future employers.
● Career Advancement: An internship or job is a significant step toward advancing your data science career. It’s a chance to gain industry-specific experience, which can lead to more specialized roles, increased responsibility, and higher positions within the field.
8. Follow and engage with the community
To thrive in the rapidly evolving field of data science, it’s essential to stay updated with the latest trends and developments. One of the most effective ways to do this is by engaging with the vibrant and dedicated data science community.
You’ll find this community active not only on mainstream social media platforms like LinkedIn, Twitter, Discord, and Reddit but also on various niche websites, insightful blogs, and influential data science leaders to follow. Seek out individuals who share your interests, don’t hesitate to reach out for advice, actively participate in discussions, and immerse yourself in the ongoing conversations.
And, if you’re looking for an excellent source of industry insights and news straight from the mouths of data professionals, be sure to tune into the DataFramed Podcast. It’s a fantastic way to stay informed and connected within the data science realm.
● Stay Informed: The field of data science is ever-evolving, much like the culinary world where new ingredients and techniques emerge. By following reputable data science blogs, websites, forums, and social media channels, you can stay up-to-date with the latest trends, tools, research, and industry news. It’s like keeping an eye on the latest food trends and culinary innovations.
● Learn from Experts: Engaging with the data science community provides you with access to experts and thought leaders in the field. You can learn from their experiences, insights, and best practices. It’s similar to learning from renowned chefs who share their culinary expertise through books, classes, and demonstrations.
● Ask Questions: Just as a budding chef might seek advice from a more experienced chef, you can ask questions and seek guidance from the data science community. Whether it’s troubleshooting a technical issue, clarifying a concept, or seeking career advice, the community is a valuable resource for getting answers.
● Networking: Engaging with the community allows you to expand your professional network. You can connect with fellow data scientists, researchers, and practitioners. These connections may lead to collaborative projects, job opportunities, or mentorship relationships.
● Contribute: Much like a chef who creates new dishes to share with the culinary community, you can contribute to the data science community. Share your knowledge, insights, and experiences through blog posts, articles, presentations, or open-source contributions. Contributing not only helps others but also establishes you as a respected member of the community.
Educational Paths to Become a Data Scientist
Becoming a data scientist is an exciting journey, and the best part is that it can kick off from various educational backgrounds. While many people start with a bachelor’s degree in fields like data science, computer science, or related areas, there are multiple pathways to acquire the necessary skills:
1. Online Courses and Certifications: Embarking on your data science journey can be as simple as diving into online resources like DataCamp’s data science tracks. These courses not only help you build expertise in various data science domains but also offer the opportunity to become a certified data professional through our recognized certification.
2. Undergraduate Degree: A solid foundation can be laid with a bachelor’s degree in data science, computer science, statistics, or a related field. This formal education provides essential knowledge and skills.
3. Master’s Degree: For those seeking specialization and advanced skills, pursuing a master’s degree can be a valuable choice. It allows you to delve deeper into the field and explore specific areas of interest.
4. Data Science Bootcamps: Data science boot camps offer intensive and focused training, enabling you to rapidly acquire practical skills. You can explore some of the top data science boot camps and alternative options in a separate article we’ve prepared.
No matter where you start, the journey to becoming a data scientist is about continuous learning and practical experience. Each path has its own advantages, and you can choose the one that aligns best with your goals and circumstances.
Final Thoughts on How Do I Become A Data Scientist
Embarking on the path to becoming a data scientist is an exciting journey filled with opportunities and rewards. In today’s world, data science plays a pivotal role in virtually every sector and industry, making data scientists more essential than ever before.
Whether you’re fueled by intellectual curiosity, enticed by the prospect of a lucrative salary, or driven to make informed decisions using data, a career in data science opens up a world of possibilities.
As we’ve explored in this comprehensive guide, there are numerous pathways to enter this dynamic field. You can opt for a formal education in computer science or statistics, or you can take a self-directed approach by enrolling in online courses, specialized tracks, and certifications like those offered by DataCamp. The journey to becoming a data scientist can be customized to suit your unique needs and preferences.
The job market for data scientists is flourishing, and it’s poised for significant growth in the years to come. This growth not only translates into more job opportunities but also a wider range of roles and specializations within the field. Whether you envision yourself as a data wrangler, a machine learning engineer, or a business intelligence analyst, there’s a niche waiting for you.
So, if you possess analytical skills, relish problem-solving, and are intrigued by the potential of data, there’s no better time than now to embark on the exciting path to becoming a data scientist. Your journey promises a world of exploration and impact.
Frequently Asked Questions (FAQs)
How do you become a data scientist from scratch?
Starting from scratch in data science may seem daunting, but self-learning is entirely possible. Begin by mastering statistics and mathematics fundamentals. Then, learn coding in Python, R, and SQL. Understand relational databases for data organization.
Develop data visualization skills for impactful presentations. Dive into big data and machine learning. Lastly, gain experience by setting goals, tackling projects, and joining data science communities. It’s a step-by-step journey to becoming a proficient data scientist.
Can you become a data scientist without a degree?
While many high-level data scientist positions prefer candidates with university qualifications, including Master’s degrees, it’s worth noting that you can enter the field without a formal degree. With determination, patience, dedication, adaptability, and a knack for data, you can carve out a career in this niche field. Your passion and commitment can open doors to opportunities in data science.
How long does it take to become a data scientist?
In our data scientist FAQs, we explain that for someone starting from scratch with no coding or math background, it typically takes 7 to 12 months of focused study to become an entry-level data scientist. But it’s important to note that data science is a field where learning continues throughout your career, with numerous skills to explore and master.
Who is suited to a career as a data scientist?
A career in data science is well-suited for individuals who are naturally curious, analytical, and have a genuine passion for information. It demands problem-solving abilities, along with perseverance and self-driven motivation.
Successful data scientists are skilled at simplifying complex issues into clear and concise explanations, making effective communication a valuable asset in this field.
What are some common tasks that data scientists perform?
A significant part of a data scientist’s job revolves around data exploration, modeling, and deployment. They typically begin by defining the problem, then gather and clean the data before drawing meaningful conclusions from the information collected. You can find more insights in our comprehensive guide on data science use cases.
What is required to become a data scientist?
Becoming a data scientist involves honing two key skills:
Skill 1: Database Mastery: You’ll need to become proficient in managing and analyzing data through databases like Oracle® Database, MySQL®, Microsoft® SQL Server, and Teradata®.
Skill 2: Statistical and Mathematical Proficiency: Another critical aspect is a solid grasp of statistics, probability, and mathematical analysis. These skills are the foundation for making data-driven decisions and insights.
How to become a data scientist?
Here’s a streamlined roadmap for becoming a data scientist:
- Earn a Data Science Degree: Begin by obtaining a degree in data science or a related field to build a strong foundation.
- Sharpen Relevant Skills: Continuously enhance your skills in areas like coding, statistics, and data analysis through online courses and self-study.
- Secure an Entry-Level Analytics Job: Gain practical experience by landing an entry-level job in data analytics or a related role.
- Prepare for Data Science Interviews: Ready yourself for data science job interviews by practicing coding challenges, honing your problem-solving abilities, and refining your communication skills.
Can I become a data scientist in 1 year?
Many individuals from diverse backgrounds, including those with no prior coding experience, have successfully transitioned into competent data scientists within just one year. The key lies in smart and focused learning, making it entirely achievable.
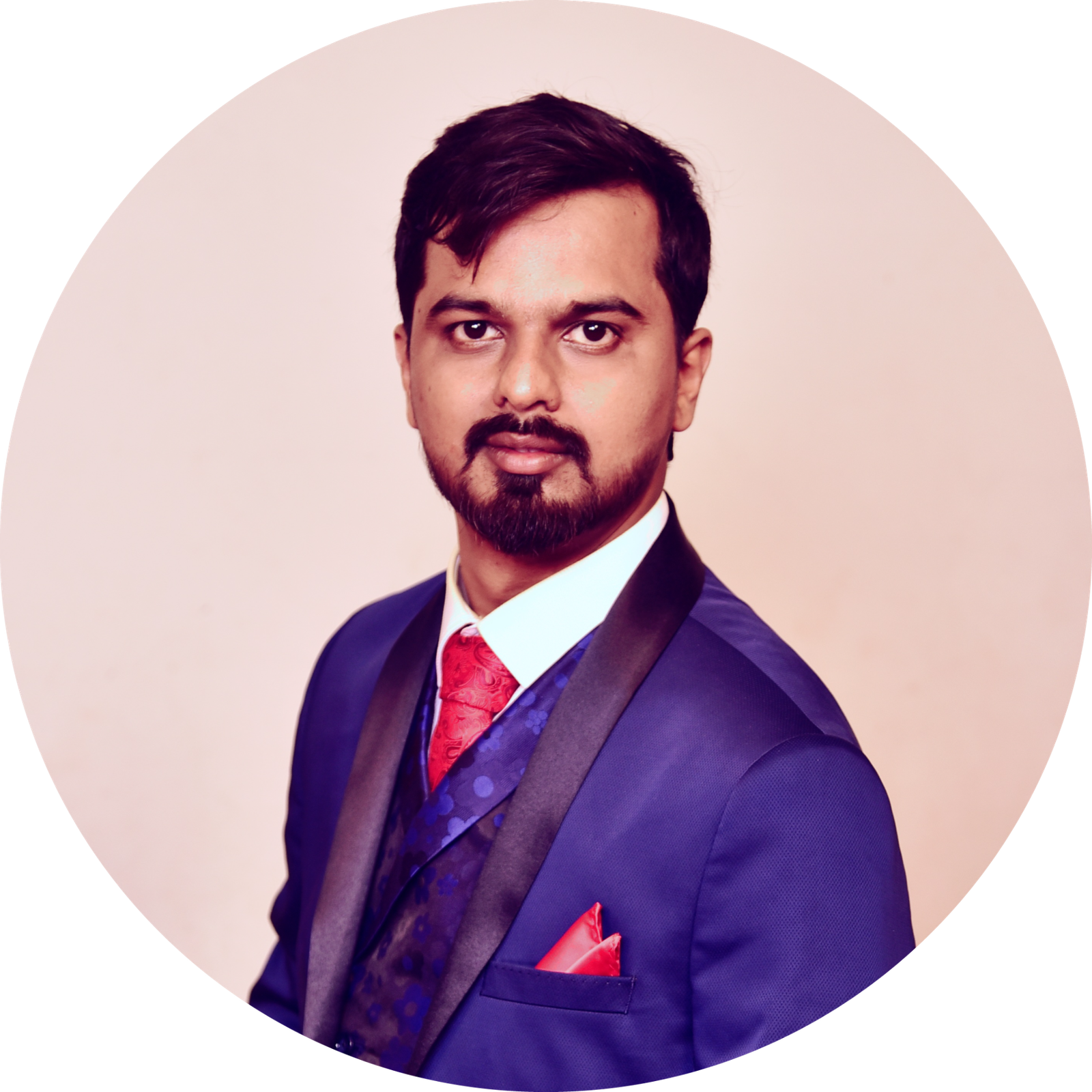
Hey, I am Sachin Ramdurg. I run and manage futuredecider.com website that helps students, graduates, and professionals, to find and decide on their future career with ultimate future career advices and future career guides. I have an overall 12+ years of career guidance experience in multiple domains which has helped multiple students, graduates, and professionals to find the best career path for their future.